
In 2014 (When I wrote my first blog on big data Link), Snowflake wasn't an outlier. AWS Redshift was just two years old, GCP was still almost a moonshot project for Google, and the list goes on. In 2021, these companies and technologies have become a behemoth on their own, with a significant market share, but the complexity of extracting value from data has drastically increased!

Extracting the value of big data is challenging because it requires a combination of highly skilled resources, technology that is fit for purpose and scales, a process that involves the business at the core.
Corporates' Growth With Big Data
Despite the often overdone buzz around big data, the underlying statement is correct: data—and data-driven decisions—now represent the next frontier of innovation and production.
Some giant corporations have reaped considerable benefits from big data by utilizing a new technology. Visa recently reported that expanding the number of characteristics it examines in each credit card transaction from 40 to 200 saved the business $6 for every $100 transaction. Wal-Mart employs a self-teaching semantic search tool that, sharpened by the monthly clickstream data of 45 million online consumers, tailors products to online customers, and has increased the percentage of completed transactions by more than 10%.
Challenges to Extracting Value From Data in SMEs
SMEs and mid-market firms may not generate volumes of data like Google or Facebook. Yet, they can use various internal and external data to understand the customer pulse, identify the trends, analyze competition, improve operational efficiency, etc.
Cleary SMEs and mid-market segments will benefit a lot by putting data to work. So the question now is not whether big data can make SMEs big, but can current data platforms make SMEs and the mid-market bigger? The answer is a BIG NO.
To understand the rationale for a "BIG NO", it is fundamental to understand the data value chain.
Data collection and ingestion
Data ingestion is the process of moving data from one or more inputs to a location where it may be stored and processed further. The data might be in many forms and come from various sources, such as RDBMS, other types of databases, S3 buckets, CSVs, or streams.
The three Vs frequently define Big Data (or just data nowadays):
Its volume
The velocity with which the data is generated and handled
The variety of data kinds includes structured and unstructured
The first two V's are self-evident; technology has enabled the acquisition of ever-increasing volumes of information and its real-time processing.
Because the data originates from many sources, it must be cleaned and converted to be analyzed alongside other sources and on time. Otherwise, the data is like a jumble of mismatched jigsaw pieces.
2. Data profiling to understand the data quality
Data profiling is the practice of analyzing existing data and summarizing information about that data. It is all about evaluating, analyzing, and synthesizing data into meaningful summaries. The method produces a high-level overview that assists in identifying data quality concerns, hazards, and general trends. Data profiling generates essential insights into data that businesses may subsequently use to their advantage.
3. Cleansing/wrangling of data with poor quality
Make an incorrect data file as well as a legitimate data file. Examine your data fields for invalid characters, such as letters in phone numbers or odd symbols in addresses.
Because this procedure is time-intensive, many firms rely on a 3rd party provider to identify and remove faulty data sets, especially before launching a new marketing campaign.
4. Data integration and pipeline building
Data integration integrates data from many sources while presenting consumers with a uniform picture of the combined data. This allows you to query and manage your data from a single interface and generate analytics, infographics, and statistics. You may also move your combined data to a different data repository for longer-term processing and storing.
A data pipeline is a collection of tools and procedures that collects data from many sources and inserts it into a data warehouse or another type of tool or application. Modern data pipelines are built to do two things: specify what, where, and how data is gathered, and automate procedures for extracting, transforming, combining, validating, and loading that data into a database, data warehouse, or app for further visualization and analysis.
5. Automation
True automation should enable the users to update data on an open data portal automatically rather than manually. Streamlining the data uploading process is critical for the long-term viability of any data initiative. Any manually updated data risks being delayed since it is yet another job that an individual must do as part of their overall workload.
6. Data governance
Big data needs strict data governance frameworks. Without them, IT systems that have not been updated to handle enormous amounts of data are likely to fail due to the sheer volume of data being processed.
The Challenges
1. Complexity and cost involved on cloud or build bespoke solutions
2. Technical skills required to build a solution on the cloud or bespoke solution
The challenge is that all the components are engineering-heavy, requiring highly skilled, highly paid, and people who are updated on new and emerging technologies.
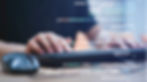
For example: In the US (July 2021 on Linkedin), there were 63K Data Engineers, 14K open roles for Data Engineering, 22% unfulfilled Data Engineering roles at any time. There were 14K Data Engineers in the UK and 1710 roles- 12% of which are unfilled. There is a massive gap between demand and supply of Data Engineers, and SMEs and mid-market can't afford to hire expensive Data Engineers.
The Solution
There are two ways to tackle this challenge. A simple solution is to increase the number of Data Engineers in the market. But at the pace of data explosion and market need, it will only become increasingly challenging to have more Data Engineers.
Quantumics.AI (https://www.quantumics.ai/) wants to solve this problem differently. We want to solve the problem by enabling the business users, i.e., to Ingest, Profile, Cleanse, Engineer (Data Pipeline), Automate, Govern and use data for analytics and AI without writing a single line of code. And we call this "Citizen DataOps."